Extracting Farm Boundaries using Segment Geospatial#
Introduction#
We use the samgeo package to automatically segment a GeoTIFF image and extract the farm boundaries as a vector layer. This is an example of zero-shot learning where we are able to extract the boundaries without providing any training samples or prompts.
Overview of the Task#
The Segment Anything model requires 3-band color imagery as the input. We take colorized image of NDVI derived from a Landsat image and use it to extract the boundaries of central-pivot irrigation farms present in the image.
Input Layers:
central_pivot_ndvi.tif
: A GeoTIFF image of NDVI computed from a Landsat-8 composite. The image has been exported from Google Earth Engine. See reference script.
Output Layers:
segment.shp
: A shapefile containing polygons of centroal-pivot irrigated farms.
Data Credit
Landsat-8 image courtesy of the U.S. Geological Survey
# Installation of segment-geospatial can take a few minutes. Please be patient
%%capture
if 'google.colab' in str(get_ipython()):
!pip install segment-geospatial rioxarray
import geopandas as gpd
import os
import matplotlib.pyplot as plt
import rioxarray as rxr
from samgeo import SamGeo
import zipfile
data_folder = 'data'
output_folder = 'output'
if not os.path.exists(data_folder):
os.mkdir(data_folder)
if not os.path.exists(output_folder):
os.mkdir(output_folder)
def download(url):
filename = os.path.join(data_folder, os.path.basename(url))
if not os.path.exists(filename):
from urllib.request import urlretrieve
local, _ = urlretrieve(url, filename)
print('Downloaded ' + local)
data_url = 'https://github.com/spatialthoughts/geopython-tutorials/releases/download/data/'
image = 'central_pivot_ndvi.tif'
download(data_url + image)
Downloaded data/central_pivot_ndvi.tif
Load the Image#
image_path = os.path.join(data_folder, image)
image = rxr.open_rasterio(image_path)
fig, ax = plt.subplots(1, 1)
fig.set_size_inches(10,5)
image.plot.imshow(ax=ax)
ax.set_axis_off()
ax.set_aspect('equal')
ax.set_title('Image')
plt.show()
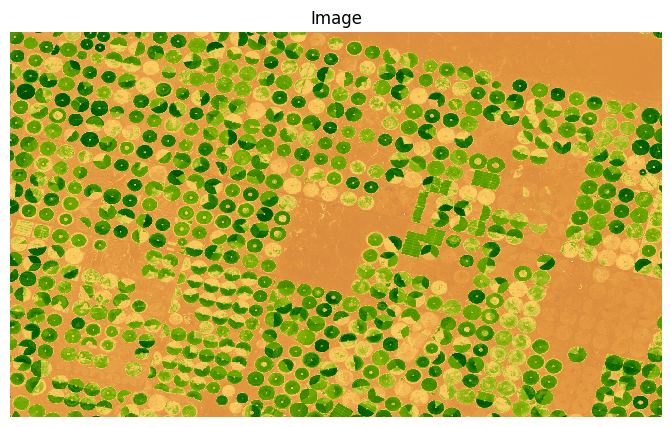
Segment the Image#
sam = SamGeo(
model_type='vit_h',
#checkpoint='sam_vit_h_4b8939.pth',
sam_kwargs=None,
)
mask = 'segment.tif'
mask_path = os.path.join(output_folder, mask)
sam.generate(image_path, mask_path, unique=True)
Polygonize the Results#
Save the segmentation results as a shapefile.
shapefile = 'segment.shp'
shapefile_path = os.path.join(output_folder, shapefile)
sam.tiff_to_shp(mask_path, shapefile_path, simplify_tolerance=None)
Visualize the results.
fig, ax = plt.subplots(1, 1)
fig.set_size_inches(10,5)
segments_gdf = gpd.read_file(shapefile_path)
segments_gdf.plot(
ax=ax,
linewidth=1,
facecolor='none',
alpha=0.5
)
ax.set_axis_off()
ax.set_aspect('equal')
ax.set_title('Extracted Segments')
plt.show()
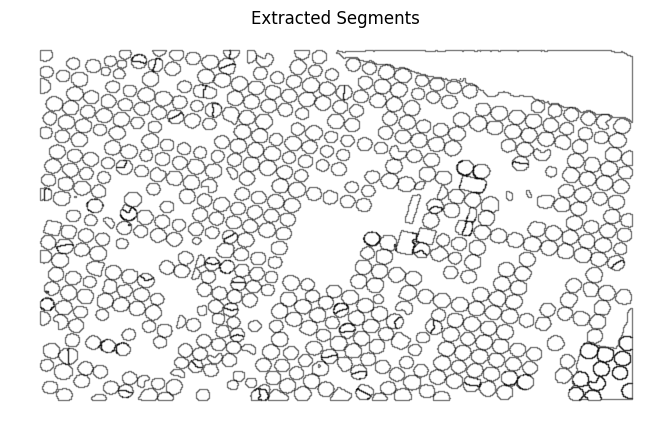
The shapefile contains farm boundaries along with background polygon covering the entire region. As we had specified unique=True
when generating the mask polygons, each polygon contains a unique id which is proporation to its area. The larger values indicate large polygons.
gdf = gpd.read_file(shapefile_path)
gdf.sort_values('value', ascending=False)
value | geometry | |
---|---|---|
127 | 630.0 | POLYGON ((38.28386 30.27098, 38.28494 30.27098... |
981 | 629.0 | POLYGON ((38.43289 30.05619, 38.43316 30.05619... |
778 | 629.0 | POLYGON ((38.44044 30.09042, 38.44071 30.09042... |
930 | 629.0 | POLYGON ((38.42723 30.06266, 38.4275 30.06266,... |
771 | 629.0 | POLYGON ((38.43774 30.09123, 38.43801 30.09123... |
... | ... | ... |
592 | 5.0 | POLYGON ((38.16582 30.13381, 38.16636 30.13381... |
228 | 4.0 | POLYGON ((38.151 30.20307, 38.15423 30.20307, ... |
485 | 3.0 | POLYGON ((38.19682 30.15591, 38.19816 30.15591... |
333 | 2.0 | POLYGON ((38.38816 30.18393, 38.38977 30.18393... |
321 | 1.0 | POLYGON ((38.40028 30.18474, 38.40163 30.18474... |
982 rows × 2 columns
We select a threshold and remove larger polygons.
threshold = 629
cleaned_gdf = gdf[gdf['value'] < threshold]
fig, ax = plt.subplots(1, 1)
fig.set_size_inches(10,5)
image.plot.imshow(ax=ax)
cleaned_gdf.plot(
ax=ax,
linewidth=1,
facecolor='none',
alpha=0.8
)
ax.set_axis_off()
ax.set_aspect('equal')
ax.set_title('Image with Extracted and Cleaned Segments')
plt.show()
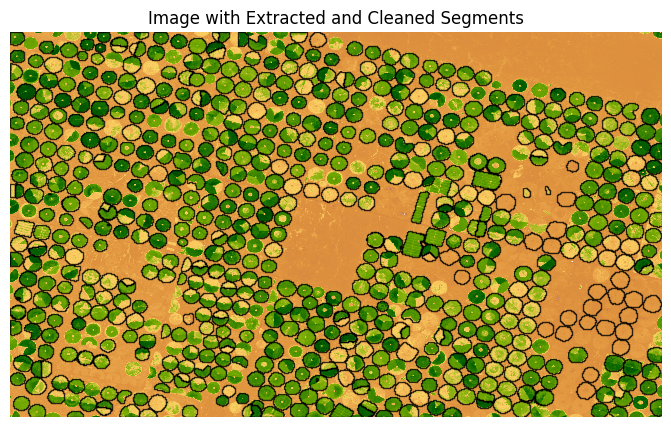
Save the results as a zipped shapefile.
edited_shapefile = 'segmentation_results.shp'
edited_shapefile_path = os.path.join(output_folder, edited_shapefile)
cleaned_gdf.to_file(edited_shapefile_path)
zipfile_name = 'segmentation_results.zip'
shapefile_base = os.path.splitext(edited_shapefile_path)[0]
zipfile_path = os.path.join(output_folder, zipfile_name)
with zipfile.ZipFile(zipfile_path, 'w') as zipf:
# Add the shapefile and its associated files
for ext in ['.shp', '.shx', '.dbf', '.prj']:
file_path = shapefile_base + ext
print(file_path)
if os.path.exists(file_path):
zipf.write(file_path, os.path.basename(file_path))
output/segmentation_results.shp
output/segmentation_results.shx
output/segmentation_results.dbf
output/segmentation_results.prj
If you want to give feedback or share your experience with this tutorial, please comment below. (requires GitHub account)